Introduction
Examples of current issues in existing datasets that impede the progress of applying AI in small molecule drug discovery:
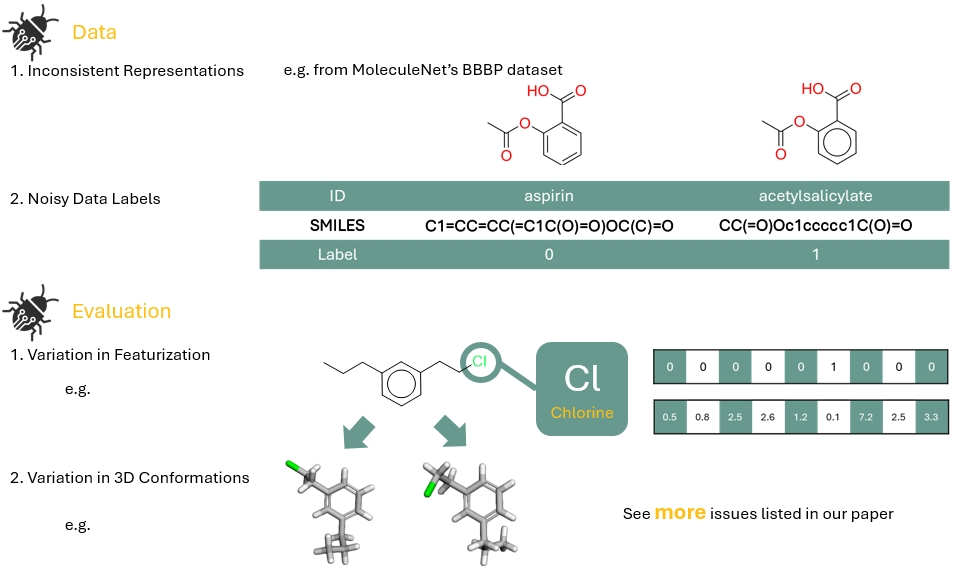
WelQrate Evaluation Framework
WelQrate Evaluation Framework establishes a foundation for benchmarking by focusing on three key aspects:
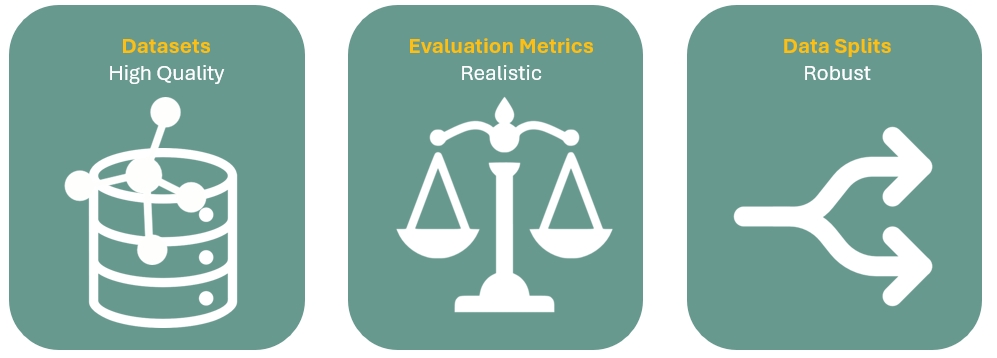
WelQrate Dataset Collection Curation Pipeline
The WelQrate dataset collection was meticulously curated by reviewing experimental data hosted on PubChem and applying multiple filters along with hierarchical curation to ensure the high quality.
(a) Curation pipeline overview
(b) An example of the hierarchical curation with AID2258.
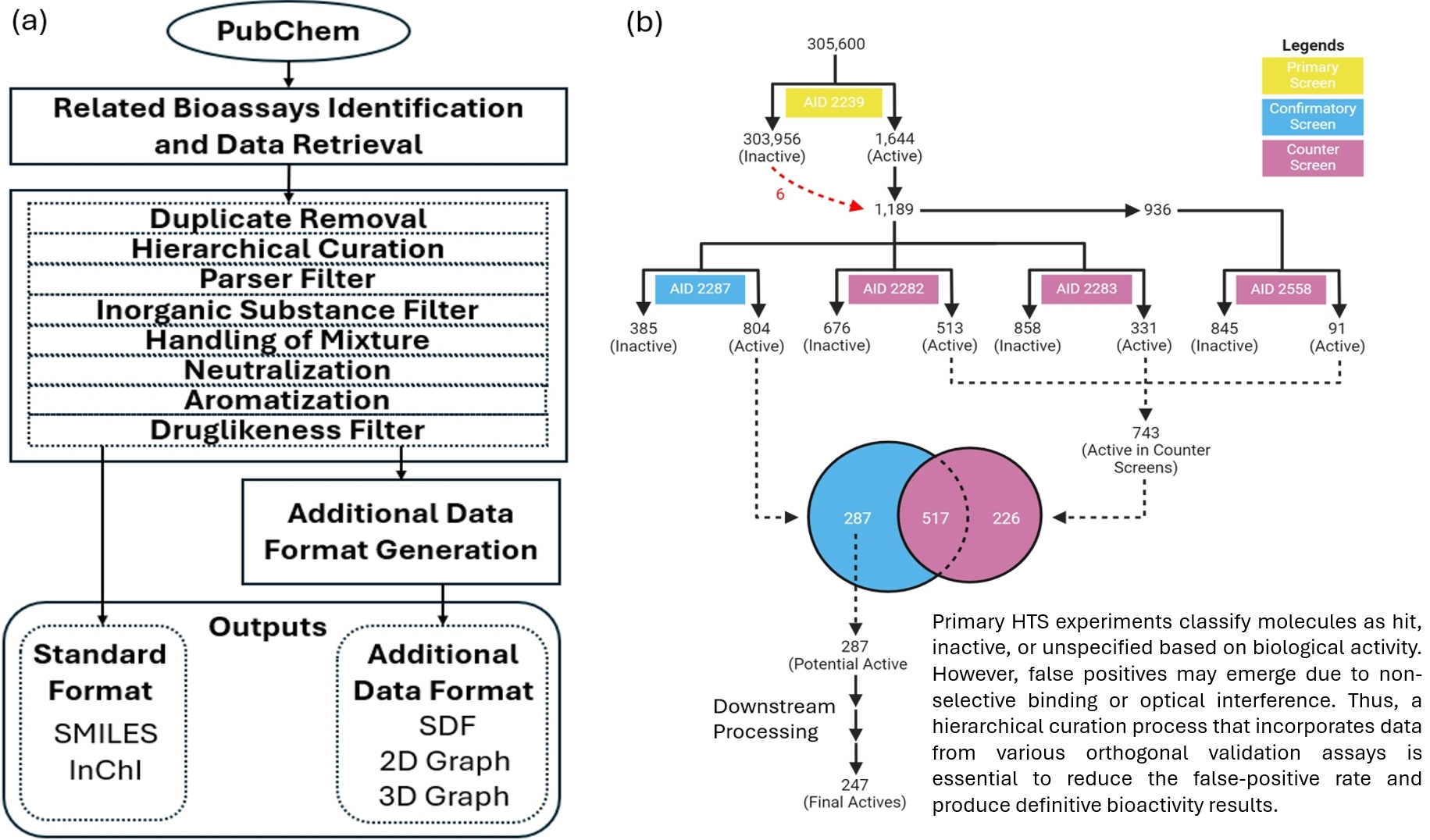
WelQrate Dataset Collection Statistics
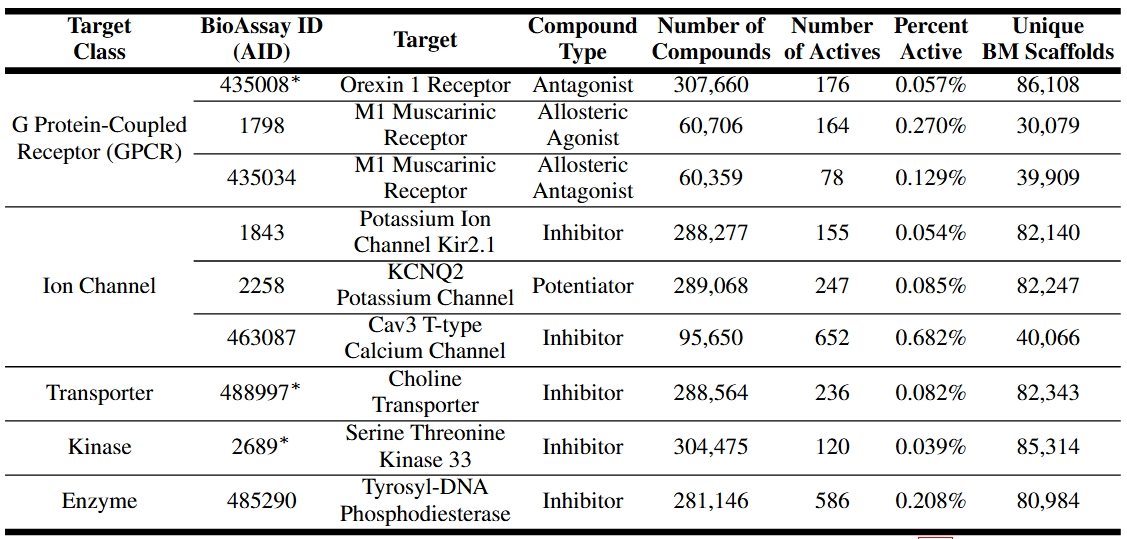
Statistics of our 9 datasets in WelQrate dataset collection, which has coverage of various important drug targets, challenging but realistic low active percentages. * Indicates additional experimental measurements are available for those datasets.
WelQrate Evaluation Metrics
In practice, only the top-ranked predicted molecules are selected for experimental validation. The WelQrate evaluation framework proposes four metrics to align with this practical approach.
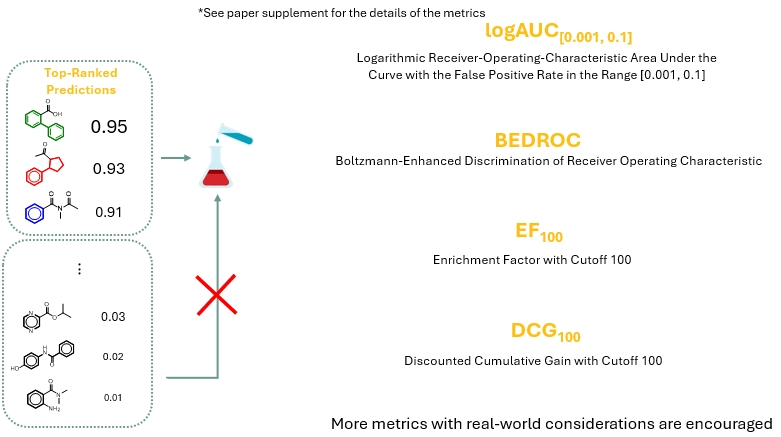
WelQrate Data Split
WelQrate provides two data split scheme, each with five different splits per dataset. Reported formance should be averaged across five splits to ensure robustness.
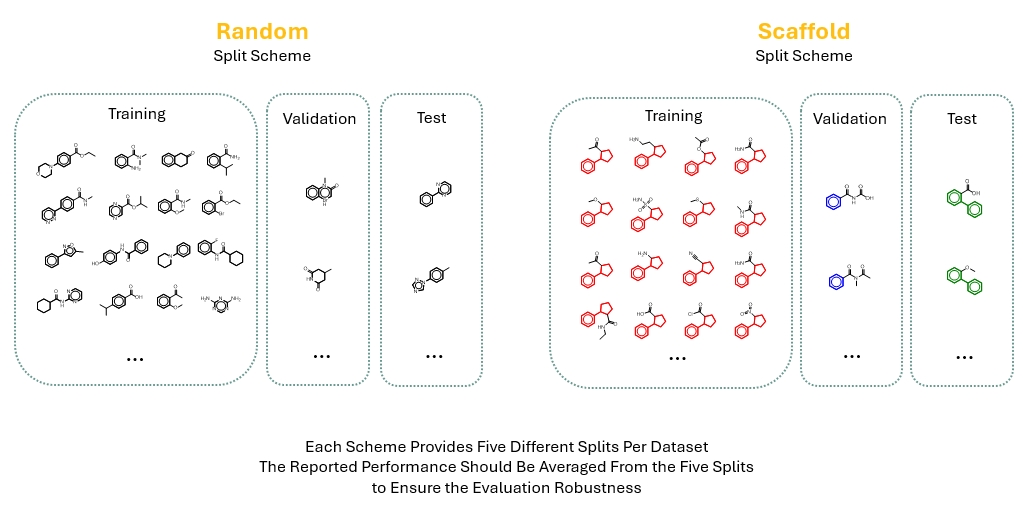
Our Team
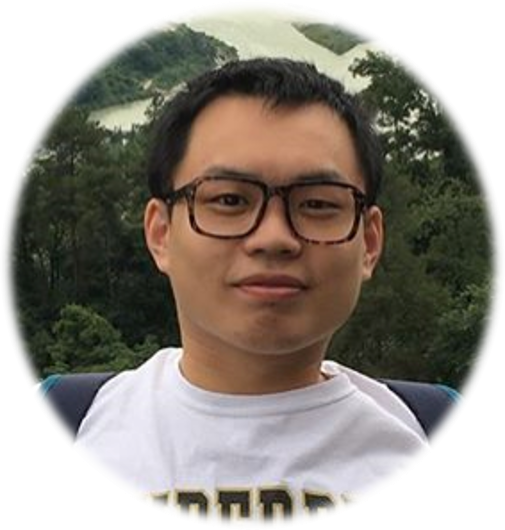
Yunchao (Lance) Liu
Vanderbilt University
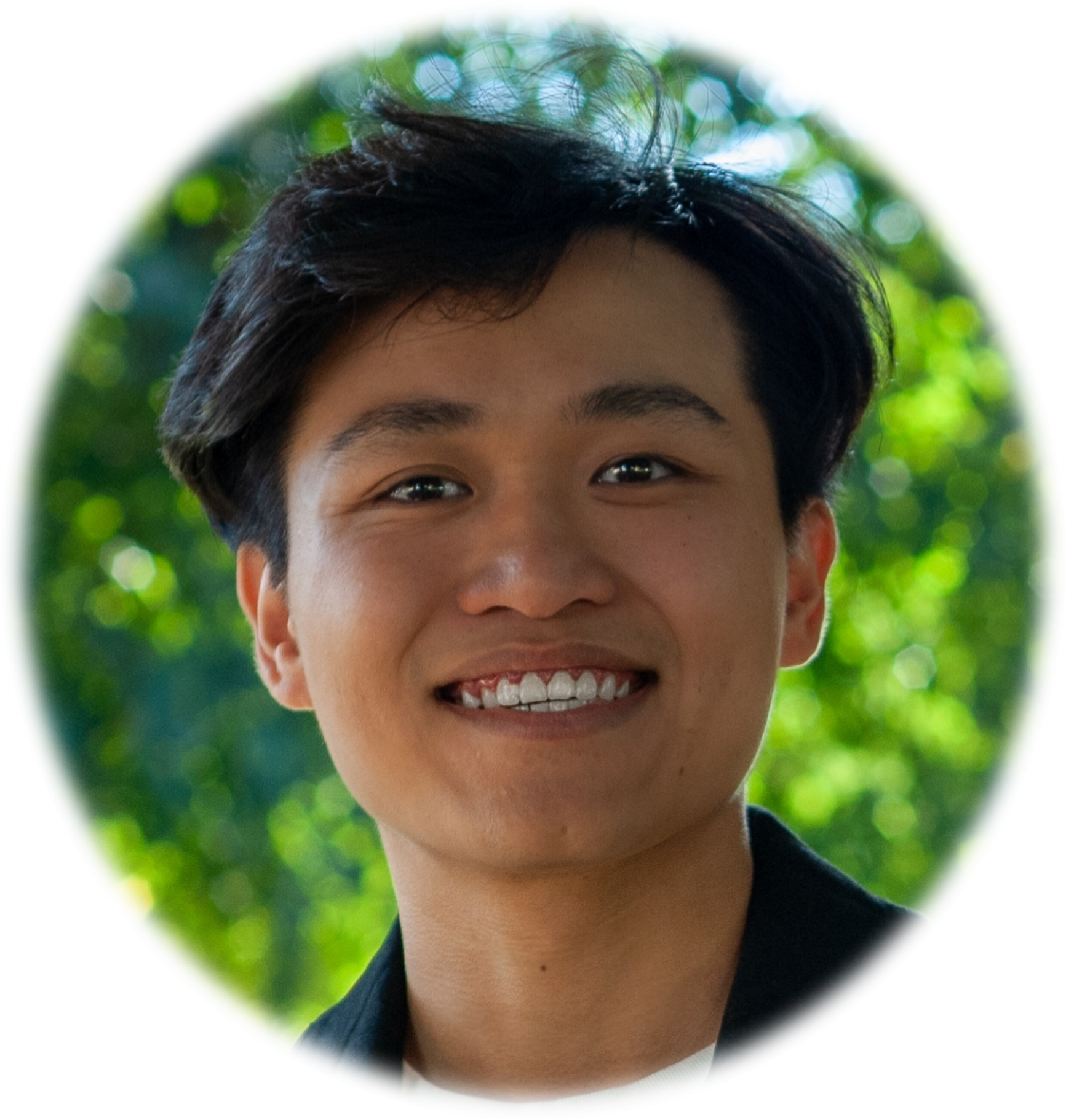
Ha Dong
Amherst College
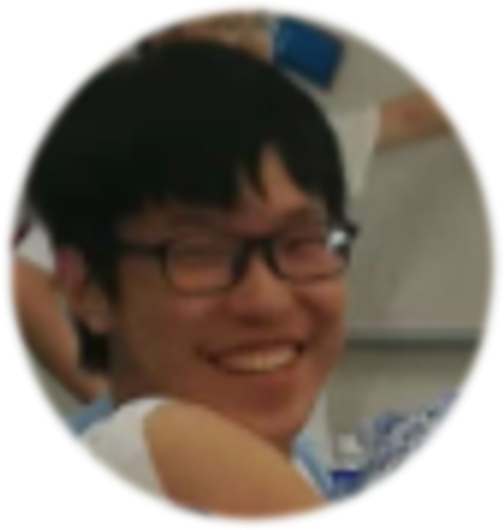
Xin (Allen) Wang
Vanderbilt University
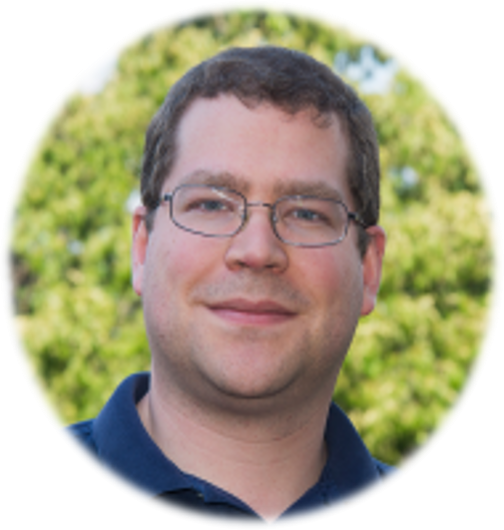
Rocco Moretti
Vanderbilt University
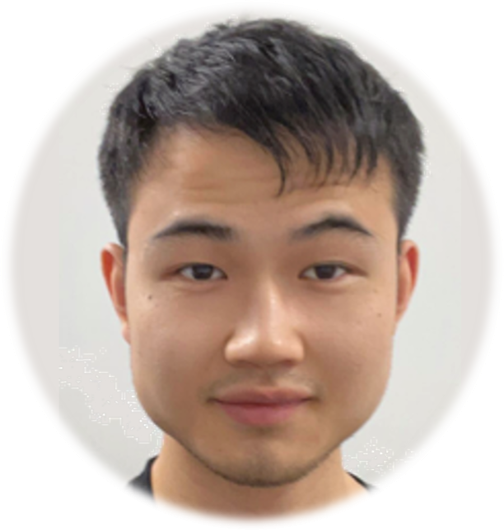
Yu Wang
University of Oregon
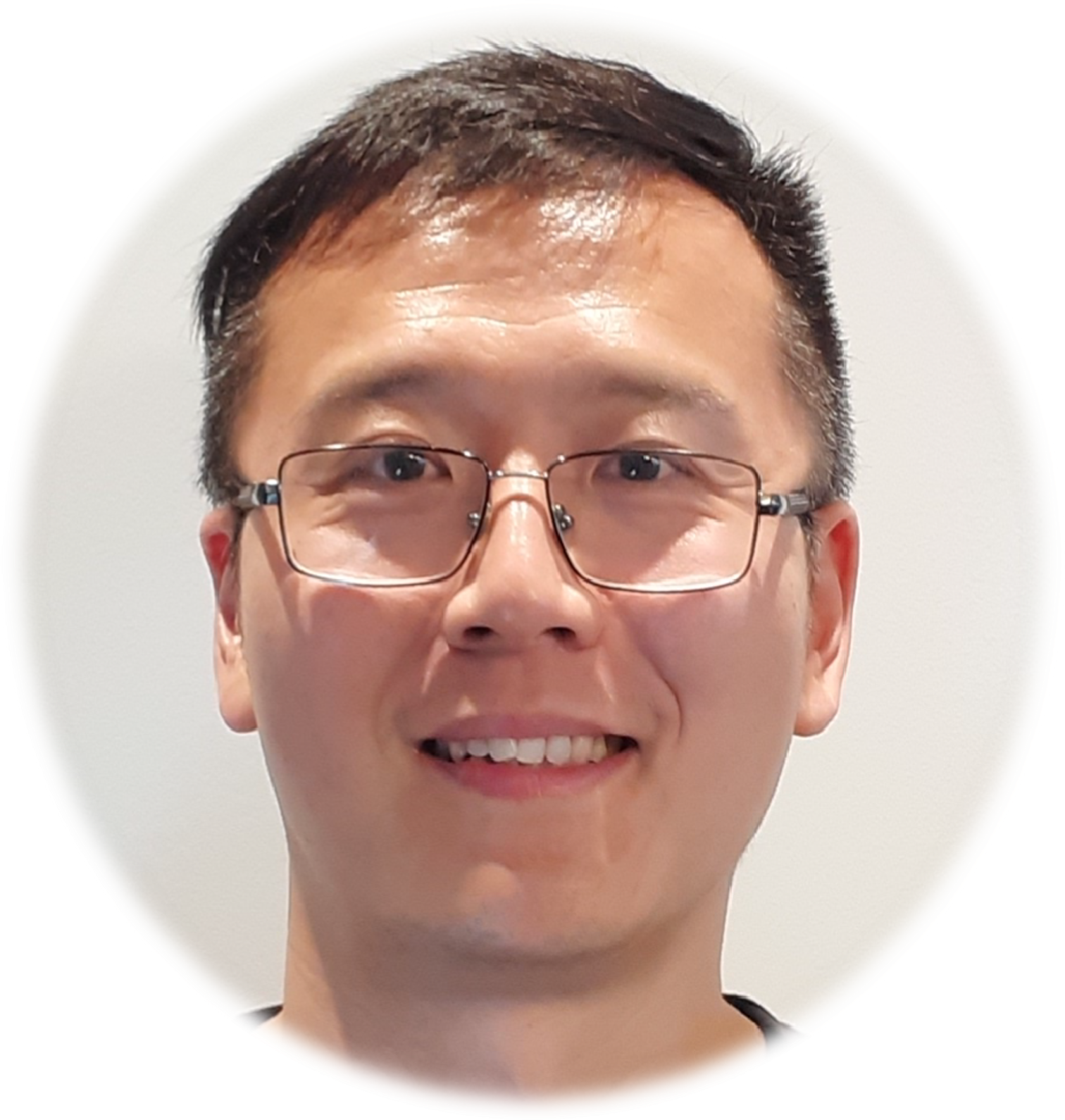
Zhaoqian (Joshua) Su
Vanderbilt University
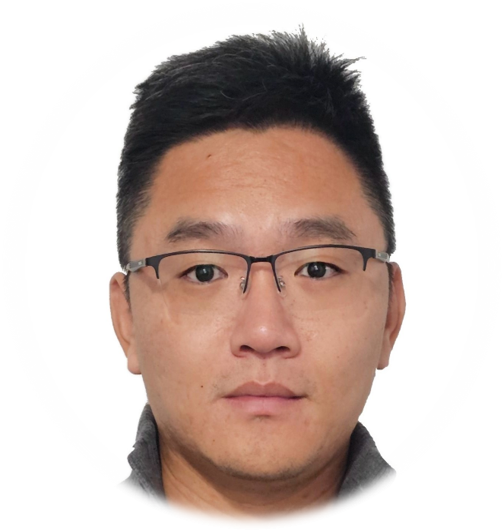
Jiawei Gu
MD Anderson Cancer Center
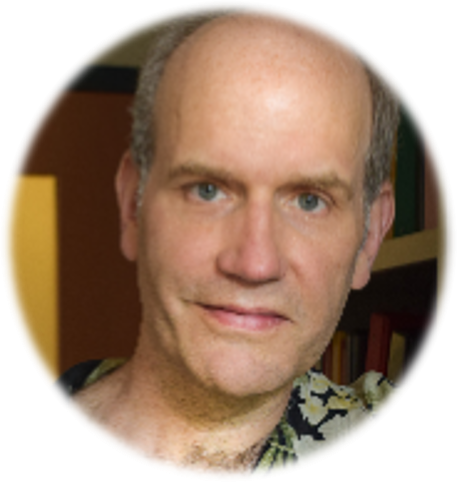
Bobby Bodenheimer
Vanderbilt University
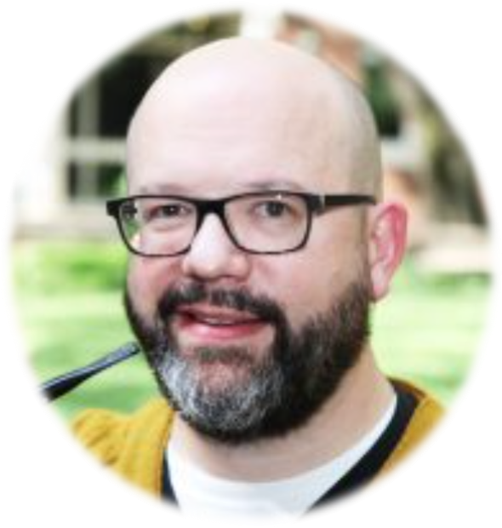
Charles David (Dave) Weaver
Vanderbilt University
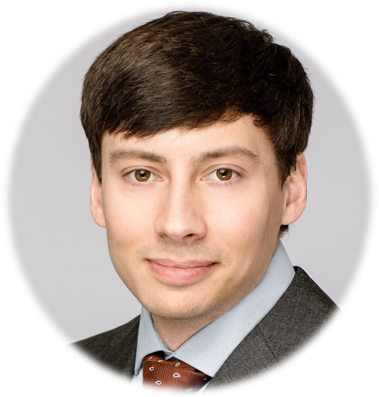
Tyler Derr
Vanderbilt University
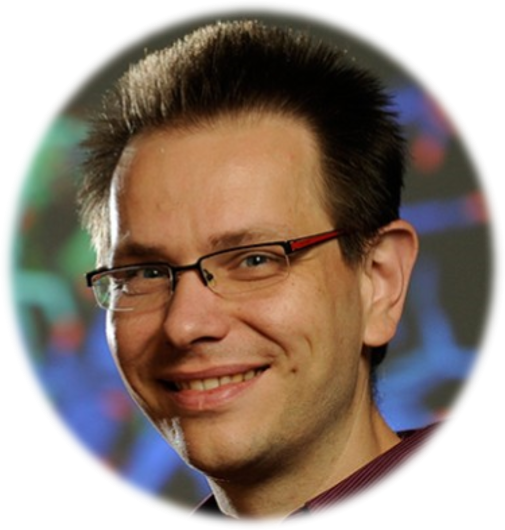
Jens Meiler
Vanderbilt University, Leipzig University